The Challenge of Trust in AI: Examining the EU’s Liability Directive
In September 2022, the European Commission unveiled a proposal for an “AI liability directive,” intending to revamp the legal landscape surrounding artificial intelligence. The core objective of this directive is to introduce a set of novel regulations within the existing European Union (EU) liability framework, specifically targeting instances where AI systems cause harm. The overarching aim is to ensure that individuals affected by AI-related incidents receive a level of legal protection commensurate with that afforded to victims of conventional technologies throughout the EU.
At the heart of this proposal is the creation of a “presumption of causality.” Essentially, it establishes a legal predisposition to attribute responsibility to AI systems when harm occurs, thus simplifying the burden of proof for those seeking recompense. Additionally, the directive empowers national courts to request the disclosure of evidence when suspicions arise regarding high-risk AI systems contributing to harm.
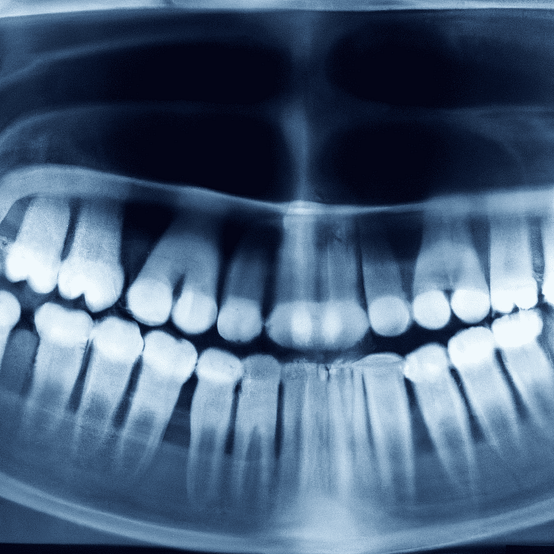
Nonetheless, this proposition is not without its detractors. Stakeholders and academics are actively scrutinizing several aspects of the proposed liability regime. Their concerns span the adequacy and efficacy of these regulations, their alignment with the concurrently negotiated artificial intelligence act, potential repercussions on innovation, and the intricate interplay between EU-level and national regulations. It is a complex legal landscape where scrutiny remains a vital component of the process.
Artificial intelligence (AI) is taking center stage in various sectors like healthcare, transportation, and agriculture, where it helps make better decisions. For instance, AI aids in diagnosing diseases, powers self-driving cars, and improves farming practices. It’s like having a digital assistant that’s helping out everywhere.
The European Commission recognizes the potential of AI but also understands the importance of keeping AI in check with EU values and principles. In their 2020 White Paper on Artificial Intelligence, they promised to take a ‘human-centric’ approach to AI. They want to make sure AI doesn’t go rogue and harm people or businesses.
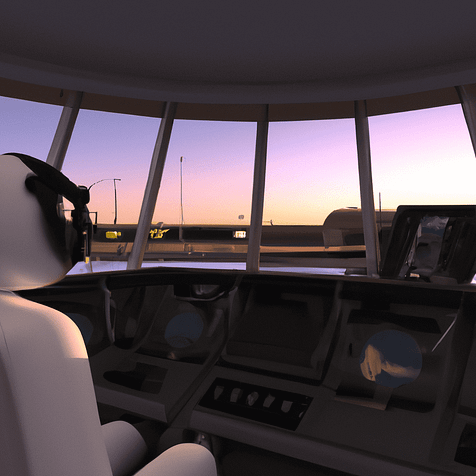
Now, when things go wrong with AI, figuring out who’s responsible and who should pay is a real puzzle. This gets even more complicated when AI teams up with the internet of things and robotics. The result? People and businesses in the EU are a bit wary of trusting AI. They like the idea of AI making their lives easier, but they’re also worried about things going south.
In fact, a recent survey in the EU found that 33% of companies feel that dealing with who’s responsible for AI-caused problems is one of the big headaches when it comes to using AI. So, there’s potential, but also some growing pains when it comes to AI adoption in the EU. It’s like having a powerful tool in your hand, but you’re not entirely sure if it’s safe to use.
It’s not just the people who use AI tools and make decisions with them who are concerned; it’s also the individuals and companies developing AI systems. They’re worried about the liability for the results their AI systems produce. As a result, most AI developers are hyper-focused on minimizing these liability risks right from the beginning, even during the early stages of planning and design. This cautious approach often results in AI systems that offer a narrow range of outputs, all carefully tailored to minimize potential risks. The goal is to create applications that are as safe as possible.
However, there’s a potential downside to this approach. When AI systems only provide answers that are absolutely foolproof and fully secure in every aspect, it might raise questions about their reliability. Trusting a system that’s overly rigid, even if it appears quite versatile on the surface, can be a bit of a conundrum. In essence, there’s a trade-off between safety and flexibility in AI development.
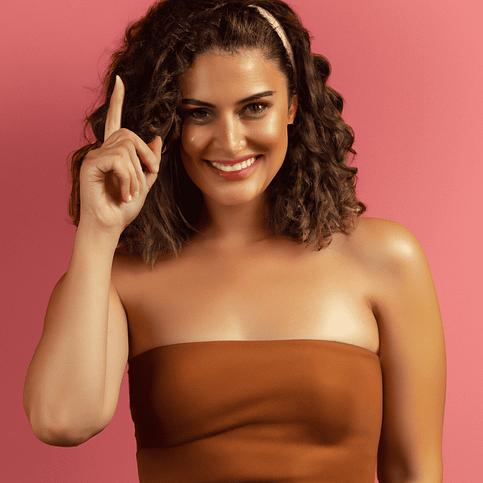
Taking this a step further, the idea of creating a completely unleashed AI without any risks to developers, prioritizing speed of development above all, could lead to a violation of human rights. This is particularly concerning if this approach places something like the “survival of mankind” as its highest value. There’s a real risk that as AI development progresses, such a requirement could be enforced, especially in authoritarian political systems.
Given these considerations, the ongoing trust dilemma involving human instincts and intuition is likely to persist for an extended period. The alternative, which entails fully embracing AI without caution, seems too bleak to gain widespread trust at this point. That’s the current outlook.
But the evolution of AI is reshaping the concept of trust. Recent scientific research suggests that trust in AI is not a static or absolute concept but a dynamic and relative one. As AI continues to advance, trust is intertwined with transparency, user experience, ethics, and human-AI collaboration. The pace of AI progress is indeed redefining our understanding of trust, and our relationship with technology, with trust becoming a multifaceted, adaptive element in this evolving landscape.